Glass Technology
Glass Melting Process
Progress of science in recent times offer greater possibilities of applying new models for the prediction and evaluation of new strategies and technologies for the furnace and glass melting processes.
In the process of manufacturing glass and glass products one of the most important step is melting the raw materials for getting glass. Mixed raw materials enter into the melting furnace.
The process of melting and purification is performed in various types and sizes of furnaces, which can work continuously and batch. Batch ovens have little capacity while the continuous furnaces have a great capacity. Continuous furnaces are more used than the batch. Type and size of the furnace depends on the amount and type of glass that is produced.
In the picture below, we show technological view of melting glass:
The process of melting is performed at about 1500 °C. This step of production is done when the glass is free of any crystalline material. In this case, a continuous furnace is used. Advanced process control of melting raw materials for glass formation in industrial furnaces is used. Oxy-fuel boosting is used to increase pull rate on a furnace that is at capacity or that has been crippled due to a failure or loss of effectiveness of the air-fuel combustion system. Oxy fuel combustion technology offers several advantages over the air technology.
The entire process of production of glass is visible on the block diagram in Figure 1.
Figure 1. Block scheme of the production process of glass.
Model Predictive Control (MPC)
The process of melting glass is inert process with large dead time. In most cases, these processes are guided by the classical PI or PID controllers. These controllers cannot achieve high quality control and therefore it is necessary to implement advanced control.
It increases the quality of glass melts and reduces fuel consumption and emissions of gas. This deficiency is particularly evident in the long furnaces to produce large quantities of glass. In such furnaces it is necessary for higher quality build as many burners. In commercial systems for process automation that are used in the process industry at the present time there are possibilities of implementation advanced control systems that can contribute to improving the quality of the production process.
For this purpose, in this case is implemented model predictive control (MPC) for process of melting the raw materials for glass formation in furnace (shown on figure 2).
Considered furnace has eight burners on one side and eight burners on the other side. Burners are placed in a zig-zag arrangement. One zone of furnace makes a couple of burners, one on the left and one on the right side.
On the burners across control valves comes natural gas and oxygen. Restrictions on commercial systems for process automation (PCS7) for model predictive control are 4 inputs and 4 outputs. In this case the furnace has 8 heating zones, and therefore, 8 controlled variables (crown temperatures).
Therefore we use two MPC controllers. One controller is used for the controlling of the first 4 zones and another for the controlling with the other 4 zones. The temperature of the fourth zone affects the temperature in the fifth zone, and the temperature of the fourth zone is taken as a disturbance at the entrance of the second MPC controller.
So these two MPC controllers are connected. Reference values of the controller are the desired temperature profile in furnace.
Models of the transfer functions are obtained by the procedure of identification on the basis of the measured response of all process variables. MPC controller as manipulated value, for each zone, gives a correction of the reference value for gas flow (ΔSP).
Corrected reference value of gas flow (SP - ΔSP) is input in PI (PID) controller for flow of gas.
The flow of oxygen and gas flow are associated with ratio that depends on the quality of natural gas and oxygen. This ratio is obtained from the analysis of gas and depends on the purity of oxygen and the amount of methane in natural gas.
Gas analysis is done in real time so the ratio changes in real time. Corrected reference value of gas flow is multiplied by a factor ratio (about 2,1) and this product represents the reference value for PI (PID) controller for flow of gas. The correct ratio of gas and oxygen is important because of the greater efficiency of energy (Economical consumption).
Figure 2. Block scheme of advanced process control in industrial glass melting furnace
This kind of model are useful in all processes where we have large death time
Neural Networks
Regulated temperatures (controlled variables) are crown temperatures. Therefore, these temperatures must be measured.
In order to achieve higher quality it is important to keep temperatures closer to the desired temperature profile.
The temperature is also measured at the bottom of each zone (bottom temperatures). Temperature probes used to measure crown temperatures are exposed to high temperatures (about 1500 °C or higher) and their lifetime is not long.
In the event that a malfunction occurs in the probe manufacturing process will be disrupted, and thus the quality of glass. In the worst case will have to stop production it will cause some losses.
To avoid such a problem in the system is installed 8 temperature soft sensors (shown on figure 3). For each crown temperature is used one soft sensor. It is derived from the static neural network of 15 inputs and 1 output. (Bottom temperature in each zone and crown temperature in each zone except those which is used).
Output is the temperature at the top of each zone resulting learned neural network. Software sensors serve as a redundant system of measurement. In case of failure on the temperature probe software switch transfers on software sensor and as controlled variable is sent on MPC controller the temperature from soft sensor.
Production can continue to run smoothly until the probe is replaced or remove the defect in it.
Figure 3. Block scheme of the temperature soft sensors
Fuzzy Logic - Disturbance values estimation
Disturbances that occur in these processes are non-measurable but have an impact on the process.
Therefore, it is implemented disturbance compensation, for each zone, using fuzzy logic, based on the experience of operators and technologists (shown on figure 4). Block for disturbance compensation has 4 inputs and 1 output. Inputs are: ambient temperature, humidity environment, amount of cullets in raw materials and regulatory tolerance in each zone. Output is the correction value acting on the exit of PI (PID) flow controller of gas in burners (Input for Control valve of gas) and at the exit of PI (PID) flow controller of oxygen in burners (Input for Control valve of oxygen), multiplied by a factor ratio. So disturbance compensation effect immediately when the disturbance occurs. Dashed part of the picture is what in the future can be implemented, but for now will be out of that part.
Figure 4. Block scheme of fuzzy logic for disturbance compensation
Progress of science in recent times offer greater possibilities of applying new models for the prediction and evaluation of new strategies and technologies for the furnace and glass melting processes.
In the process of manufacturing glass and glass products one of the most important step is melting the raw materials for getting glass. Mixed raw materials enter into the melting furnace.
The process of melting and purification is performed in various types and sizes of furnaces, which can work continuously and batch. Batch ovens have little capacity while the continuous furnaces have a great capacity. Continuous furnaces are more used than the batch. Type and size of the furnace depends on the amount and type of glass that is produced.
In the picture below, we show technological view of melting glass:
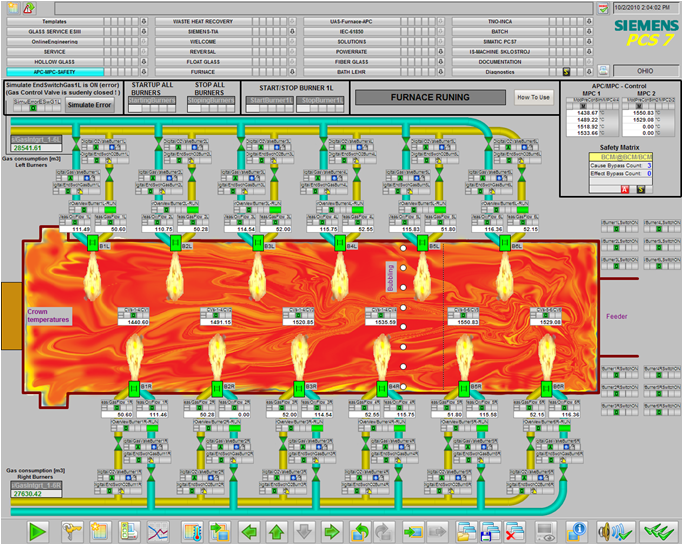
The process of melting is performed at about 1500 °C. This step of production is done when the glass is free of any crystalline material. In this case, a continuous furnace is used. Advanced process control of melting raw materials for glass formation in industrial furnaces is used. Oxy-fuel boosting is used to increase pull rate on a furnace that is at capacity or that has been crippled due to a failure or loss of effectiveness of the air-fuel combustion system. Oxy fuel combustion technology offers several advantages over the air technology.
The entire process of production of glass is visible on the block diagram in Figure 1.
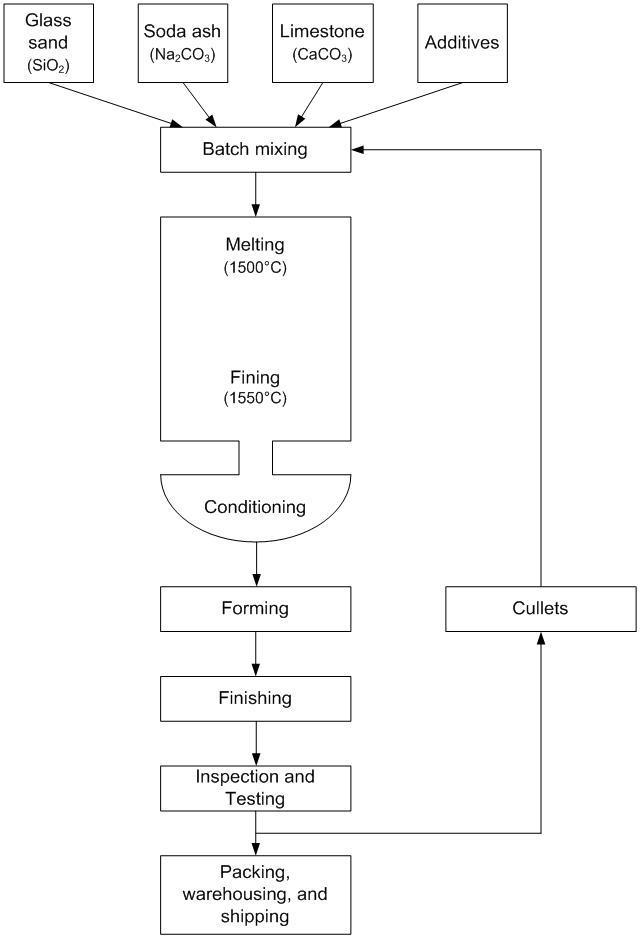
Figure 1. Block scheme of the production process of glass.
Model Predictive Control (MPC)
The process of melting glass is inert process with large dead time. In most cases, these processes are guided by the classical PI or PID controllers. These controllers cannot achieve high quality control and therefore it is necessary to implement advanced control.
It increases the quality of glass melts and reduces fuel consumption and emissions of gas. This deficiency is particularly evident in the long furnaces to produce large quantities of glass. In such furnaces it is necessary for higher quality build as many burners. In commercial systems for process automation that are used in the process industry at the present time there are possibilities of implementation advanced control systems that can contribute to improving the quality of the production process.
For this purpose, in this case is implemented model predictive control (MPC) for process of melting the raw materials for glass formation in furnace (shown on figure 2).
Considered furnace has eight burners on one side and eight burners on the other side. Burners are placed in a zig-zag arrangement. One zone of furnace makes a couple of burners, one on the left and one on the right side.
On the burners across control valves comes natural gas and oxygen. Restrictions on commercial systems for process automation (PCS7) for model predictive control are 4 inputs and 4 outputs. In this case the furnace has 8 heating zones, and therefore, 8 controlled variables (crown temperatures).
Therefore we use two MPC controllers. One controller is used for the controlling of the first 4 zones and another for the controlling with the other 4 zones. The temperature of the fourth zone affects the temperature in the fifth zone, and the temperature of the fourth zone is taken as a disturbance at the entrance of the second MPC controller.
So these two MPC controllers are connected. Reference values of the controller are the desired temperature profile in furnace.
Models of the transfer functions are obtained by the procedure of identification on the basis of the measured response of all process variables. MPC controller as manipulated value, for each zone, gives a correction of the reference value for gas flow (ΔSP).
Corrected reference value of gas flow (SP - ΔSP) is input in PI (PID) controller for flow of gas.
The flow of oxygen and gas flow are associated with ratio that depends on the quality of natural gas and oxygen. This ratio is obtained from the analysis of gas and depends on the purity of oxygen and the amount of methane in natural gas.
Gas analysis is done in real time so the ratio changes in real time. Corrected reference value of gas flow is multiplied by a factor ratio (about 2,1) and this product represents the reference value for PI (PID) controller for flow of gas. The correct ratio of gas and oxygen is important because of the greater efficiency of energy (Economical consumption).
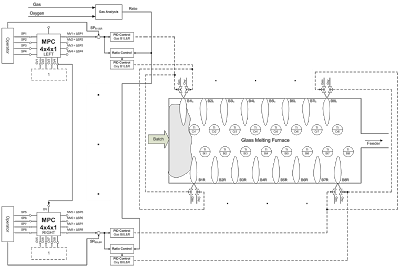
Figure 2. Block scheme of advanced process control in industrial glass melting furnace
This kind of model are useful in all processes where we have large death time
Neural Networks
Regulated temperatures (controlled variables) are crown temperatures. Therefore, these temperatures must be measured.
In order to achieve higher quality it is important to keep temperatures closer to the desired temperature profile.
The temperature is also measured at the bottom of each zone (bottom temperatures). Temperature probes used to measure crown temperatures are exposed to high temperatures (about 1500 °C or higher) and their lifetime is not long.
In the event that a malfunction occurs in the probe manufacturing process will be disrupted, and thus the quality of glass. In the worst case will have to stop production it will cause some losses.
To avoid such a problem in the system is installed 8 temperature soft sensors (shown on figure 3). For each crown temperature is used one soft sensor. It is derived from the static neural network of 15 inputs and 1 output. (Bottom temperature in each zone and crown temperature in each zone except those which is used).
Output is the temperature at the top of each zone resulting learned neural network. Software sensors serve as a redundant system of measurement. In case of failure on the temperature probe software switch transfers on software sensor and as controlled variable is sent on MPC controller the temperature from soft sensor.
Production can continue to run smoothly until the probe is replaced or remove the defect in it.
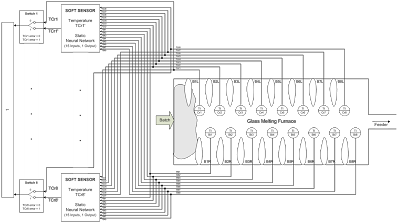
Figure 3. Block scheme of the temperature soft sensors
Fuzzy Logic - Disturbance values estimation
Disturbances that occur in these processes are non-measurable but have an impact on the process.
Therefore, it is implemented disturbance compensation, for each zone, using fuzzy logic, based on the experience of operators and technologists (shown on figure 4). Block for disturbance compensation has 4 inputs and 1 output. Inputs are: ambient temperature, humidity environment, amount of cullets in raw materials and regulatory tolerance in each zone. Output is the correction value acting on the exit of PI (PID) flow controller of gas in burners (Input for Control valve of gas) and at the exit of PI (PID) flow controller of oxygen in burners (Input for Control valve of oxygen), multiplied by a factor ratio. So disturbance compensation effect immediately when the disturbance occurs. Dashed part of the picture is what in the future can be implemented, but for now will be out of that part.
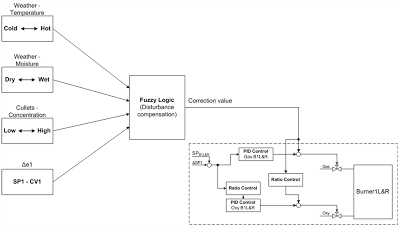
Figure 4. Block scheme of fuzzy logic for disturbance compensation